The Welding Research Laboratory at the University of Kentucky is focused on system identification, adaptive and predictive control, neurofuzzy modeling and control, machine vision systems, and various sensors with applications to welding/manufacturing automation, especially to the development of innovative electrical arc welding processes with improved productivity/controllability. The Welding Research Laboratory projects include advanced sensing, control, learning/modeling, and intelligent robotic systems with application to real-time monitoring and control of innovative welding processes, human welder modeling, human welder centered control, human-robot collaborative systems, and intelligent welding robots as gained from efforts supported by the NSF, Navy, National Labs and industry with a total funding of $7.5 M.
Current research thrusts of the Welding Research Lab are focused primarily on:
- Learning and Modeling of Human Welder Response Behaviors for Intelligent Welding Robots
- Deep Learning of Reflection Pattern due to Weld Pool Oscillation for Intelligent Monitoring and Control of Weld Joint Penetration
- Learning of Complex Human Welder Intelligence from Big Data Generated from Augmented and Virtual Reality Welding Systems
- Analytic Weld Pool Model Calibrated by Measurements with Application to Real-Time Monitoring and Control of Invisible Weld Pool underneath the Work-piece
The research interests:
- Automation of industrial processes
- Welding processes
- Numerical modeling of welding processes
- Adaptive control systems, robust control theory and systems, dynamic process identification
- Machine vision, signal processing
Director: Dr. Yuming Zhang
Projects
Qiang Ye, YuMing Zhang, Qiang (Shawn) Cheng. “RI: Small: Optimal Transport Generative Adversarial Networks: Theory, Algorithms, and Applications,” National Science Foundation, IIS-2327113, $590,000, October 1, 2023-September 30, 2026.
Y.M. Zhang, P. Wang. “NRI: FND: Intelligent Co-robots for Complex Welding Manufacturing through Learning and Generalization of Welders Capabilities,” National Science Foundation, CMMI-2024614, $665,540, August 1, 2020-July 31, 2023. No cost extension to July 31, 2024.
Publications
- Rui Yu, Yue Cao, Jennifer Martin, Otto Chiang, and YuMing Zhang, 2024. Robotizing Double-Electrode GMAW Process through Learning from Human Welders. Journal of Manufacturing Processes, 109: 140-150.
- Rui Yu, Yue Cao, Heping Chen, Qiang Ye, YuMing Zhang, 2023. “Deep Learning Based Real-Time and In-Situ Monitoring of Weld Penetration: Where we are and what are needed revolutionary solutions?” Journal of Manufacturing Processes, 93 (2023) 15-46, DOI 10.1016/j.jmapro.2023.03.011
- Edison Mucllari, Rui Yu, Yue Cao, Qiang Ye, YuMing Zhang, 2023. “Do We Need a New Foundation to Use Deep Learning to Monitor Weld Penetration?” IEEE Robotics and Automation Letters, 8(6): 3669 – 3676, DOI 10.1109/LRA.2023.3270038.
- Rui Yu, Yue Cao, Jennifer Martin, Otto Chiang, YuMing Zhang, 2023. Deep-Learning Based Supervisory Monitoring of Robotized DE-GMAW Process Through Learning from Human Welders. Topic Collection of Intelligent Welding Manufacturing, Welding in the World. DOI:10.1007/s40194-023-01635-y
- Rui Yu, Joseph Kershaw, Peng Wang, YuMing Zhang, 2022. “How to accurately monitor the weld penetration from dynamic weld pool serial images using CNN-LSTM deep learning model?,” IEEE Robotics and Automation Letters, VOL. 7, NO. 3, JULY 2022, 6519-6525, DOI 10.1109/LRA.2022.3173659.
- Heping Chen, Ahmed Yaseer, Yuming Zhang, 2022. “Top Surface Roughness Modeling for Robotic Wire Arc Additive Manufacturing,” Journal of Manufacturing and Materials Processing, 2022, 6, 39. https://doi.org/10.3390/jmmp6020039.
- Peng Wang, Joseph Kershaw, Matthew Russell, Jianjing Zhang, YuMing Zhang, Robert X. Gao, 2022. “Data-driven process characterization and adaptive control in robotic arc welding,” CIRP Annals, 71(1), pp.45-48.
- YuMing Zhang, Qiyue Wang, Yukang Liu, 2021. Adaptive Intelligent Welding Manufacturing – Classical Sensing/Modeling/Control and Modern Machine Learning and Human-Robot Collaborative Approaches. Welding Journal, 100(2): 63s-83s, https://doi.org/10.29391/2021.100.006.
- Qiyue Wang, Wenhua Jiao, Peng Wang, YuMing Zhang, 2021. Digital Twin for Human-robot Interactive Welding and Welder Behavior Analysis. IEEE/CAA Journal of Automatica Sinica, 8(2): 334-343, DOI: 10.1109/JAS.2020.1003518
- Wenhua Jiao, Qiyue Wang, Yongchao Cheng, YuMing Zhang, 2021. End-to-end prediction of weld penetration: A deep learning and transfer learning based method. Journal of Manufacturing Processes, Volume 63, March 2021, pages 191-197, https://doi.org/10.1016/j.jmapro.2020.01.044
- Qiyue Wang, Wenhua Jiao, Peng Wang, YuMing Zhang, 2021. A tutorial on deep learning-based data analytics in manufacturing: Through a welding case study. Journal of Manufacturing Processes, Volume 63, March 2021, pages 2-13, https://doi.org/10.1016/j.jmapro.2020.04.044.
- Yongchao Cheng, Shujun Chen, Jun Xiao, YuMing Zhang, 2021. Dynamic estimation of joint penetration by deep learning from weld pool image. Science and Technology of Welding and Joining, 26(4): 279-285, https://doi.org/10.1080/13621718.2021.1896141
- YC Cheng, QY Wang, WH Jiao, J. Xiao, SJ Chen, YM Zhang, 2021. Automated Recognition of Weld Pool Characteristics from Active Vision Sensing. Welding Journal, 100(5): 183s-192s, https://doi.org/10.29391/2021.100.015
- Cheng, Y., Yu, R., Zhou, Q., Chen, H., Yuan, W. and Zhang, Y., 2021. Real-time sensing of gas metal arc welding process–A literature review and analysis. Journal of Manufacturing Processes, 70, pp.452-469.
- Joseph Kershaw, Rui Yu, YuMing Zhang, and Peng Wang. Hybrid Machine Learning-Enabled Adaptive Welding Speed Control. Journal of Manufacturing Processes, 71 (2021): 374-383.
- Rui Yu, Joseph Kershaw, Peng Wang, YuMing Zhang. Real-Time Recognition of Arc Weld Pool Using Image Segmentation Network. Journal of Manufacturing Processes, 72(2021): 159-167
- YuMing Zhang, Yu-Ping Yang, Wei Zhang, Suck-Joo Na, 2020. Advanced Welding Manufacturing - A Brief Analysis and Review of Challenges and Solutions. J. Manuf. Sci. Eng., Special Issue: MED’s 100th Anniversary, 142(11), Article number 110816, https://doi.org/10.1115/1.4047947
- Qiyue Wang, Wenhua Jiao, YuMing Zhang, 2020. Deep learning-empowered digital twin for visualized weld joint growth monitoring and penetration control. Journal of Manufacturing Systems, 57: 429–439, October 2020, Pages 429-439https://doi.org/10.1016/j.jmsy.2020.10.002
- Chunyang Xia, Zengxi Pan, Joseph Polden, Huijun Li, Yanling Xu, Shanben Chen, and Yuming Zhang, 2020. A review on wire arc additive manufacturing: Monitoring, control and a framework of automated system. Journal of Manufacturing Systems, October 2020, 57: 31-45, https://doi.org/10.1016/j.jmsy.2020.08.008
- Wenhua Jiao, Qiyue Wang, Yongchao Cheng, Rui Yu and YuMing Zhang, 2020. Prediction of Weld Penetration Using Dynamic Weld Pool Arc Images. Welding Journal, 99(11): 295s-302s, https://doi.org/10.29391/2020.99.027
- Chao Li, Qiyue Wang, Wenhua Jiao, Michael Johnson, YuMing Zhang, 2020. Deep Learning-Based Detection of Penetration from Weld Pool Reflection Images. Welding Journal, 99(9): 239s-245s, https://doi.org/10.29391/2020.99.022
- Yongchao Cheng, Qiyue Wang, Wenhua Jiao, Rui Yu, Shujun Chen, YuMing Zhang, Jun Xiao, 2020. Detecting Dynamic Development of Weld Pool Using Machine Learning from Innovative Composite Images for Adaptive Welding. Journal of Manufacturing Processes, 56, Part A, August 2020, pp. 908-915, https://doi.org/10.1016/j.jmapro.2020.04.059.
- Qiyue Wang, Wenhua Jiao, Rui Yu, Michael T. Johnson, YuMing Zhang, 2020. Virtual Reality Robot-Assisted Welding Based on Human Intention Recognition. IEEE Transactions on Automation Science and Engineering, 17(2): 799-808, DOI: 10.1109/TASE.2019.2945607
- Qiyue Wang, Yongchao Cheng, Wenhua Jiao, Michael T. Johnson, YuMing Zhang, 2019.
- Virtual Reality Human-Robot Collaborative Welding: A Case Study of Weaving Gas Tungsten Arc Welding. Journal of Manufacturing Processes, Volume 48, December 2019, Pages 210-217, https://doi.org/10.1016/j.jmapro.2019.10.016
- J.S. Chen, J. Chen, Z.L. Feng, Y.M. Zhang, 2019. Model Predictive Control of GTAW Weld Pool Penetration, 2019. IEEE Robotics and Automation Letters, 4(3): 2762 – 2768, DOI: 10.1109/LRA.2019.2918681
- Qiyue Wang, Wenhua Jiao, Rui Yu, Michael Johnson, YuMing Zhang, 2019. Modeling of Human Welder Operation in Virtual Reality Human-Robot Interaction Welding System. IEEE Robotics and Automation Letters, 4(3): 2958-2964, DOI: 10.1109/LRA.2019.2921928.
- Zhimin Liang, Hexi Chang, Qiyue Wang, Dianlong Wang, YuMing Zhang, 2019. 3D Reconstruction of Weld Pool Surface in Pulsed GMAW by Passive Biprism Stereo Vision. IEEE Robotics and Automation Letters, 4(3): 2377-3766, DOI: 10.1109/LRA.2019.2924844.
- R. Liang, R. Yu, Y. Luo, Y.M. Zhang, 2019. Machine Learning of Weld Joint Penetration from Weld Pool Surface Using Support Vector Regression. Journal of Manufacturing Processes, 41(2019): 23-28, https://doi.org/10.1016/j.jmapro.2019.01.039
- X.Q. Yu, J.K. Huang, L. Shao, Y.M. Zhang, D. Fan, Y.T. Kang, F.Q. Yang, 2019. Microstructures in the joint of zirconium-based bulk metallic glass and copper. Materials Research Express, 6(2), Article Number: 026511, DOI: 10.1088/2053-1591/aaebf2
Facilities
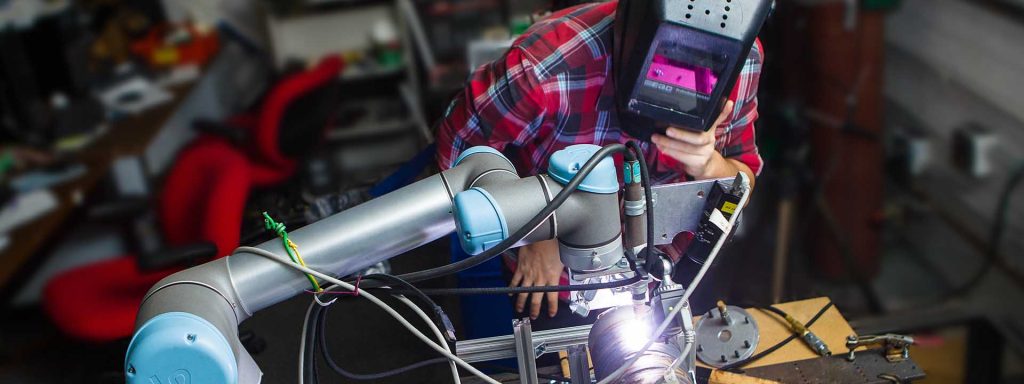
Cooperative Robot
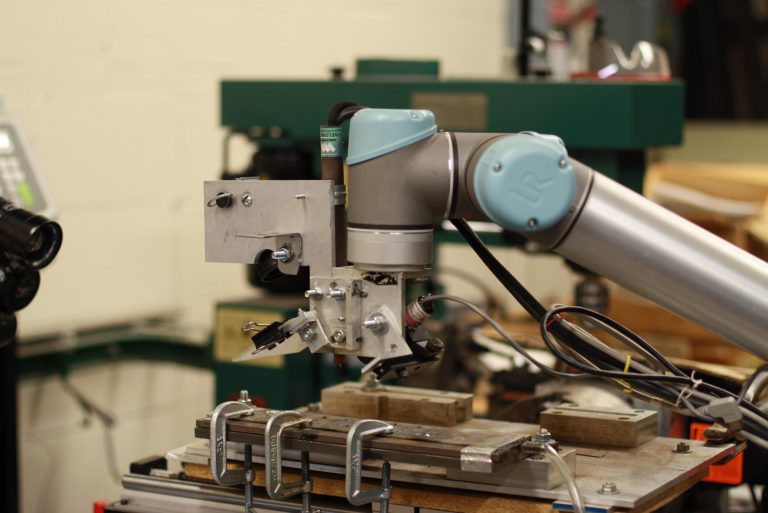
Cooperative Robot
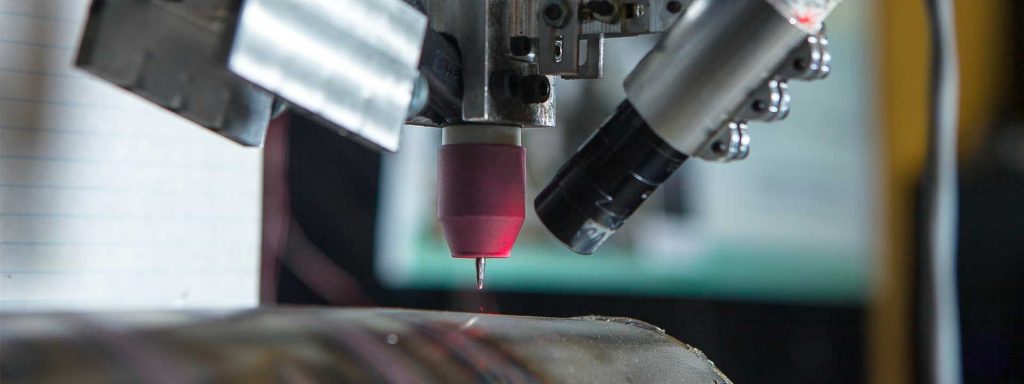
Weld Poor Surface Sensing system
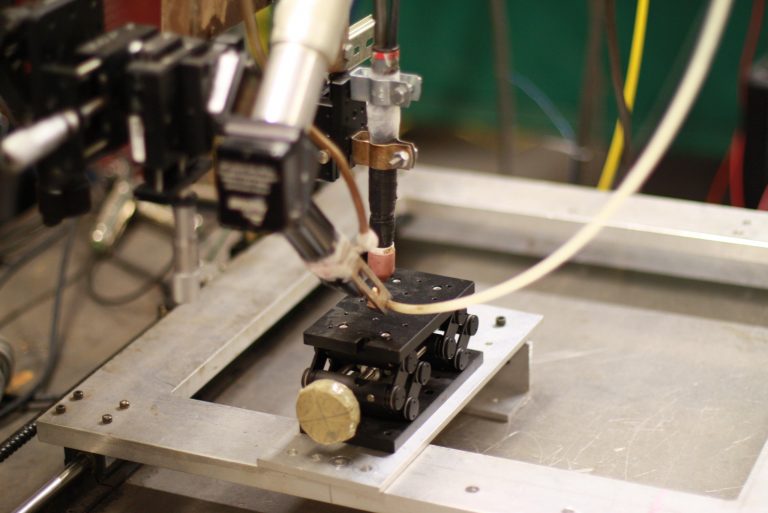
Weld Poor Surface Sensing system
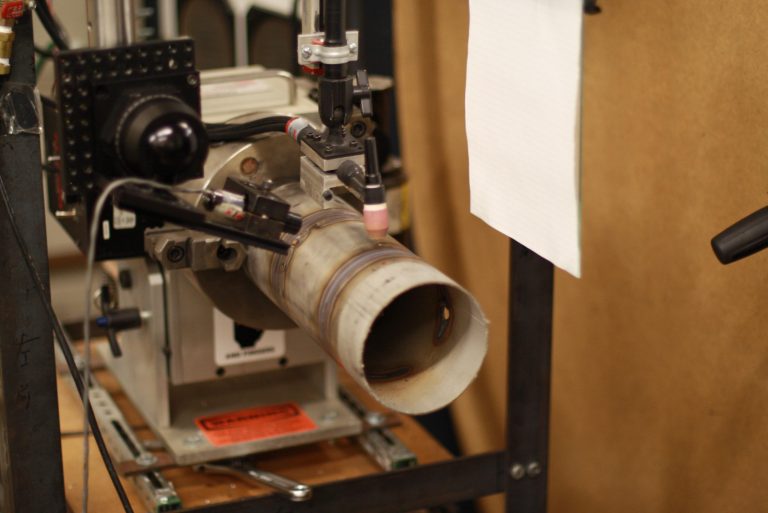
Machine Human Cooperative Welding System
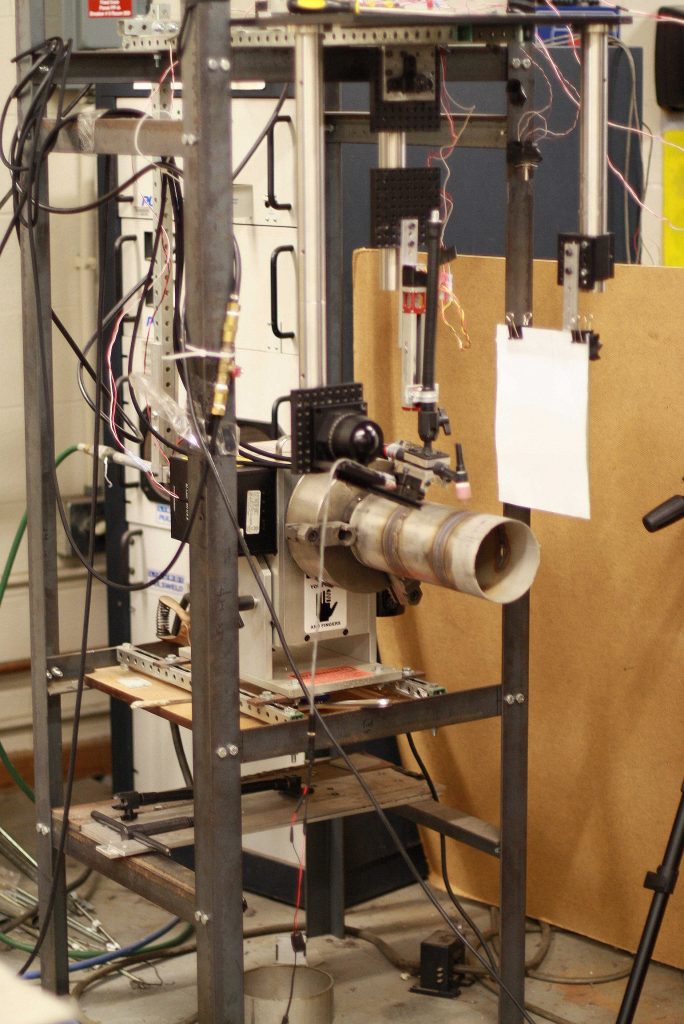
Machine Human Cooperative Welding System